Cancer is a complex and uncontrollable beast that mutates and changes continuously even before you get into the politics and the economics surrounding the issue. There are actually more than 100+ different forms of verified cancerous diseases. We all have cancer cells in our bodies, but our immune system can fight them off. Problems arise when cancer cells are triggered by certain external and internal factors, and they begin to multiply rapidly overwhelming our immune system.
Despite billions pouring in to fight cancer, we still don’t have a real cure for cancer other than a probability of survival. New discoveries about the onset and behavior of cancer are being made all the time, and they are leading to the development of more effective therapies such as vaccines, immunotherapy products, and targeted therapies, all causing fewer side effects compared with chemotherapy and radiation. However, despite this exhaustive effort, the number of cancer cases is estimated to increase by 70% over the next two decades, and is expected to reach globally 25 million new cases per year by 2030.
While the world would undoubtedly be better off without cancer, big pharmas probably not, because they stand to lose billions when it is cured. Numbers vary but in 2015 the spending on cancer medicines was around $107 billion worldwide, and this number will swell to $150 billion in 2020. Don’t get me wrong, drug companies are not withholding the secret that cures cancer, those kind of conspiracy stories have just a poetic truth. The reality is that, a lack of innovation throughout the entire process of drug development coupled to various technical artifacts, have left humanity with no cancer blockbusters drugs.
According to CB Insights, equity investment in cancer-therapeutics startups has grown from $2bn in 2013 to $4.5bn in 2017. And it’s not just big pharma that’s taken notice: tech companies such as Google and IBM have entered this space recently, investing millions into startups developing immune-oncology and targeted therapy treatments. All cancer drugs made up fully 31% of all drug programs studied (drug development)and have overall success (FDA approval) rates 5.1%.Furthmore,the entire process of drug development takes too much time (10 to 15 years) and $2.6 billion to bring a cancer drug to the market, all indicating that a big dose of digital transformation is needed. In fact, many of the analytical processes involved in drug development can be made more efficient with artificial intelligence, macchine learning and blockchain and this has the potential to shave off years of work and hundreds of millions in investments.
Artificial Intelligence and how to tame the beast
AI is predicted to change health care by advancing clinical research and drug development. Besides cutting costs, improving trial quality, and reducing trial times by almost half, AI is finding cancer biomarkers and gene signatures, recruiting eligible clinical trial patients in minutes and reading volumes of text in seconds. Moreover breakthrough discoveries involving new diagnostic tools for cancer have seen AI as a major player. Right now hundreds of startups and corporate unicorns are all working to find new diagnostic tools and treatments for cancer using AI in drug development and clinical research in all different areas:
- Diagnosis: digital pathology and medical imaging
Boston Massachusetts-based PathAI, supplies AI-powered research tools and services for digitizing and analyzing pathology images. And it’s working to make safer and more affordable the sub-typing of diseases like breast cancer. Deep Lens (USA), is extending one of the world’s first digital pathology cloud platforms that for over ten years has allowed pathology groups to collaborate on cancer research across dozens of cancer types. Proscia also makes digital pathology software and AI applications for cancer diagnosis.Inspirataprovides a cancer diagnostics solution that digitizes and automates the pathology workflow using a unique, “solution-as-a-service” delivery model. Nucleai (Israel) aims to improve cancer diagnostics making it accurate, effective, accessible and efficient in order to assist pathologists by using machine learning, deep learning, and machine vision technology.
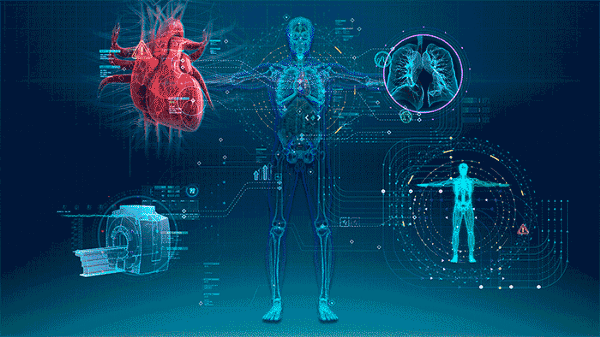
Merantix is a Germany-based AI research and incubator lab, building machine learning companies in various fields. MX Healthcare, one of its spinoffs, has developed an AI algorithm that can analyze mammogram x-rays and detect irregularities and signs of cancer with reliable accuracy. MX Healthcare has trained its AI model on more than a million mammograms from partnering radiology offices and hospitals. The company ultimately aims to develop a cloud-based, on-demand platform that will put its cancer-detection AI at the disposal of radiologists across the world. Aidence, an Amsterdam-based startup has developed its first solution, a medical image analysis software based on deep learning. This Veye Chest is already deployed in 10 hospitals and helps radiologists detect and report pulmonary nodules on a CT chest image to detect lung cancer.
Earth Scan aims to take advantage of the technology used to reliably transfer data between the Earth and spacecrafts to create a system that can improve detection rates for bowel cancer. This technology will be used by Earth Scan to link up a cloud-based AI system that can support doctors when identifying cancer in patients. This cloud-based AI will help to identify and characterise polyps by analysing a live colonoscopy video regardless of patient-doctor location.
The list of the AI diagnostic companiesis long andthey all share a common problem or challenge preventing them for reaching their full potential:the quality of the data they use. AI can interpret image scans or pathology images only if the data fed to the system is good quality data and in a large amount. Hence, if the quality of data is poor, AI systems will generate inaccurate and biased results. Moreover AI performed on retrospective pathology studies — using information on events that have taken place in the past — can also has its limitations. Try now to imagine a human organ scanned for cancer as a movie made up of 100 frames, with each frame being a singlestatic image of the whole organ, in this time series movie we call lifespan of an organ. Pathologists and their AI use fixed (chemically modified) slides (pathology images), that rappresent only one microscopic part (i.e. one part out of 1000), of one only single frame (i.e. frame 6, time=6) in order to detect cancer. Radiologists on the other hand and their AI are using the entire single frame (i.e. frame 6, time=6) — made up of all 1000 microscopic parts (6i, 6ii,…, 6m). So in order to have a very intelligent AI we will have to train it with all the microscopic parts (1000) of all single frames (1, 2, 3, …,100) of an organ, and this is what the brain does when monitoring the health of our internal organs. So in the near future or we will have to build a “Prêt à Porter AI platform” that mimics our brain for monitoring our internal organs or we will have to build an AI that simply “chats telepathically” with our brain while our brain monitors our internal organs…and I guess that future doesn’t seem too far away.
- Blood test screening
As the old saying goes “prevention is better than cure”, and for that reason the cancer prevention company Freenome (USA) is building an AI platform for screening blood to identify also future cancers. Most cancer detection methods focus on detecting the existence of cancer, but Freenome’s early cancer detention screening goes one step further by identifying where the bad tissue is likely to be-located, it also looks for markers to determine whether it may be malignant or benign (colorectal cancer) and is not limited to look for mutations instead it detects changes in gene expression, immune activity, and cancer-related proteins among billions of circulating cell-free biomarkers.
- Drug development
A drug molecular discovery focused company advancing AI technology is Atomise, usingdeep learning to analyze molecules through simulation that eliminates time taken by researchers to synthesize and test compounds. Atomwise is running over 50 different molecular discovery programs and screens up to 10 million compounds each day to discover molecules that help prevent diseases while also studying their toxicity and their reaction with the human body.
For faster cancer drug target identification and validation GlaxoSmithKline (GSK) formed a collaboration with a Baltimore-based AI-driven companyInsilico Medicine, to explore how Insilico’s AI capability can help in the identification of novel biological targets and pathways of interest to GSK. Insilico Medicine has an in silico drug discovery process described as a closed feedback loop containing stages like data mining, hypothesis generation, lead compound identification and optimization. GSK also signed a $43 M drug discovery collaboration with U.K.-based AI-driven startup Exscientia to identify small molecules for ten selected targets across undisclosed therapeutic areas. Numerate is another data driven drug design company applying AI to drug discovery. Numerate agreed to lead discovery programs for identifying clinical candidates in Takeda’s core therapeutic areas of oncology. Structure-based drug discovery (SBDD) approach complemented by the use of AI (deep learning) technology is the primary focus of a partnership between Korean biopharmaceutical company CrystalGenomics and AI-driven technology company Standigm aiming at discovering and developing novel drugs to treat cancer and liver related diseases.
All these AI drug discovery companies are all facing a bigger challenge than the AI image screening companies do. They have to screen trillions of different molecules testing them on hundreds of different types of human cells (200 different types of human cells) expressing hundreds of different types of receptors and other proteins, not to mention that every human is different and human cells still evolve. Considerations on the number of possible molecules to test has led to the concept of the “chemical space” (known and unknown) to describe the ensemble of all organic molecules to be considered when searching for new drugs.
Whereas the known chemical space including public databases and corporate collections probably contains 100 million molecules (table 1), it has been estimated that the unknown virtual chemical space might contain as many as 10⁶⁰ (novemdecillion) compounds when considering only basic structural rules, or a more modest 10²⁰–10²⁴ molecules if combination of known fragments are considered. In comparison the number of sand grains on Earth is about 7.5 x 10¹⁸. These estimations suggest that this entire chemical space is far too large for an exhaustive enumeration, even using today’s computers. One is therefore left with a partial, targeted enumeration as the only option to produce molecules for virtual screening.
- Clinical Trial
Deep 6 AI, uses AI to mine medical records to accelerate finding and recruiting patients for clinical trials within minutes. In an early comparison, Deep 6 AI’s software found and validated 58 eligible matches in less than 10 minutes while a principal investigator — using traditional recruitment methods — found 23 eligible patients in six months for a biomarker for a non-small cell lung cancer trial. Deep 6 AI, uses Natural Language Processing (NLP) to read doctors’ notes, pathology reports, diagnoses, recommendations, and to detect hard-to-find lifestyle data, such as smoking and activity history. American technology company NVIDIA is using AI in creating a common discovery platform for cancer called CANDLE, and goals include uncovering the genetic DNA and RNA of common cancers, predicting how patients will respond to treatments, how each patient’s cancer evolves, building a database of disease metastasis and recurrence, and getting new therapies to market faster.
The list of these AI clinical trial companiesis long and are all facing three major challenges: 1) accessing patient data, 2) accessing good quality data, and 3 ) creating industry-wide data standards. These standards need to include patient data in the broadest possible sense and from a wide range of sources including mobile devices, wearables and more — from healthy populations and not just cancer patients and anywhere on earth. This last challenge is likely to grow more urgent with the expected rise of the Internet of Things, when millions of devices — from factory tools to kitchen appliances — will soon be equipped with internet-connected sensors. So all kindy of data (even clinical) will be overlaid with a sensor’s geographic location, which can then be used to help identify and understand spatial patterns, behaviours and life style.
- Analysing research literature, publications, and patents. Data mining (biomedical, clinical and patient data)
A research collaboration between pharmaceutical giant Pfizer and IBM’s Watson for Drug Discovery to tackle immuno-oncology became one of the most covered news story of AI application in biopharma sector. This collaboration was aimed to bring the power of AI-driven supercomputer for accelerating analysis and tests of hypotheses by researchers at Pfizer using “massive volumes of disparate data sources” that include more than 30 million sources of laboratory data reports as well as medical literature. UK-based BenevolentAI is also using AI for scientific data mining, data contextualization and deriving hypotheses. A new scientific paper is published every 30 seconds and there are 10,000 updates to PubMed everyday, and navigating through all this information to draw meaningful insights about drug candidates is where BenevilentAI’s based algorithms become indispensable. Bio-Modeling Systems (BMSystems) is a French company, developing Computer-Assisted Deductive Integration drug discovery platform (CADI)™ based on heuristic non-mathematical models to generate novel hypotheses from scientific, medical & health data. The platform includes several components for data acquisition & mining, data organization & structuring, an integrative engine, and model representation & visualization tool. Concerto HealthAI will collaborate with Pfizer using Concerto’s eurekaHealth platform, AI models and Real World Clinical Electronic Medical Record (EMR) and healthcare claims. Concerto has an exclusive license to utilize data from 1) clinical medical practices that participate in the American Society of Clinical Oncology’s CancerLinQ initiative and others throughout the U.S. and 2) numerous other real-world sources, which might explain why big pharma companies are so eager to work with Concerto. XtalPi, NuMedii, Berg Health, Aicure, Biovista, Cloud Pharmaceuticals, Recursion Pharmaceuticals and GNS Healthcare are also among the world’s top 20 AI drug development companies.
To ensure AI pays dividends, all AI pharma companies will need to overcome apart the technological challenges also the “human challenges”. The progress of all pharma AI companies has been hindered by anxiety over change, such as the ethics of AI, and employee concerns over potential job losses. A recent survey found 67% of workers are worried about machines taking work away from people. But these fears over robots taking our jobs are misplaced. AI is just another tool that will augment researchers by helping them to tackle repetitive, time-consuming work, allowing them to be more creative and follow different paths of fruitful research.
Epilogue
The synergy of humans and AI in healthcare for finding hidden and unintuitive patterns in vast amounts of data in ways that no human can do, will definitely help us find and reach the unexplored corners of pharma for finding new treaments. This synergistic relationship that has just started will pave the way for pharma’s digital transformation, trying to tame the beast of cancer and data, while humans and computers will collaborate in a manner that will leverage the strengths of both just by learning.